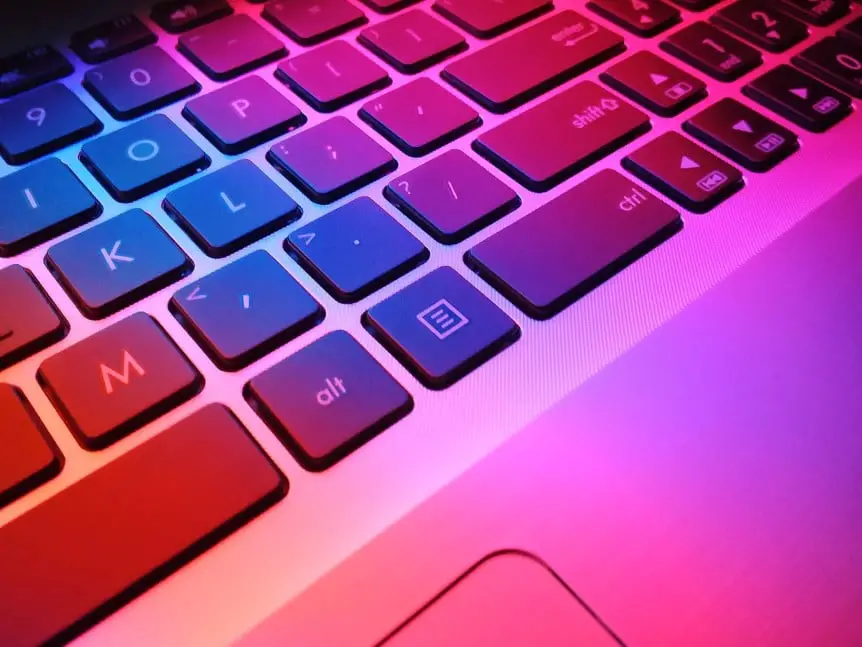
Python is cool, no doubt about it. But, there are some angles in Python that are even cooler than the usual Python stuff.
Here you can find 100 Python tips and tricks carefully curated for you. There is probably something to impress almost anyone reading it.
This is more like a Python Tricks Course that you can benefit from at various levels of difficulty and experience.
Holy Python is reader-supported. When you buy through links on our site, we may earn an affiliate commission.
Estimated Time
Flexible
Skill Level
All
Python Tips
100+
Content Sections
Beginner
1-35
Intermediate (link)
36-70
Advanced (link)
71-100
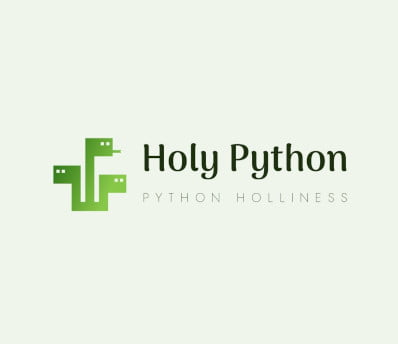
So, you might want to pace yourself to avoid burnout, skim it as you wish or go through each point if you’re a beginner.
You can also blend it with your #100daycoding #100daywithPython routines.
Tips are divided in 3 sections, this should make navigation a bit easier.:
- Beginner (tips 1 – 35)
- Intermediate (tips 36 – 70)
- Advanced (tips 71 – 100)
To make them even more manageable now, I split Intermediate & Advanced tips to separate blog posts which you can access via links. Enjoy.
Who Is It For?
If you took a Python course these somewhat niche methods might have escaped the curriculum. Or if you’re switching to Python from another language you might not have heard of these unique approaches even as a seasoned programmer.
Learning additional Python tips and tricks might give you new ideas, open new doors, make you more competent in various situations and even expand your creativity.
These Python tricks are aimed at a programming audience at varying levels. Here are some ideas who might benefit from the list:
- If you took a Python course lately
- If you recently started learning Python
- If you are still learning Python and got the basics under control
- If you are an experienced programmer but switching to Python for the first time
- If you are curious about some interesting and unique ways you can craft your code in Python
- If you are an advanced user but still suspecting you might be missing out on tricks you’ve never seen before.
Feel free to digest these in chunks, combine with your #100dayPython routine, review at different periods or before Python job interviews.
Here are 100 Python Tips and Tricks, starting with the beginner tips:
Python Tips & Tricks (Part I)
Beginner Level
HolyPython.com
If you are dedicated to learning Python and creating great things in the future with it. This list will offer you a great push to cover some of the topics that can go unnoticed or not be included in the conventional Python lessons.
The list includes a few well known Python concepts as well as lots of lesser known tricks and even touches on computer science a little bit in the next session.
We hope you enjoy the full list and if you have time share the love on social media.
Let’s start with beginner Python tips!
1) print(\a): (Make print sing)
Okay, technically not singing but can you believe printing \a creates a Windows chime?
This is because \a is reserved to warning notification sound in Windows and printing it in Python triggers it.
You can check out this post to see an alarm clock made by Python’s print function only.
import antigravity
2) Dictionary Merge: (Python Syntax Show Off)
Here is a fantastic Python approach. Do you have 2 dictionaries that you’d like to merge easily? You can use ** notation for **kwargs-like objects (values with names like dictionaries) to merge them conveninently.
Let’s say you have 2 Olympics results from 2 separate countries that held the events. Dictionary 1 named: China_olympics and then Japan_olympics and you’d like to make a shallow merge. All you have to do is:
{**China_olympics, **Japan_olympics}
Python will take care of the rest, making it a breeze.
Here is a demonstration:
d1={"A": 10, "B": 20, "C": 30}
d2={"X": 100, "Y": 200, "Z": 300}
d3={**d1, **d2}
print(d3)
{'A': 10, 'B': 20, 'C': 30, 'X': 100, 'Y': 200, 'Z': 300}
3) Proper File Paths: (Handling Paths)
r''
This one is a life saver. standing for raw string, r in front of the string quotes ensure that file paths don’t get confused between Python and system settings.
Whenever you’re typing paths in your code, just by including that r in front of the quotes you can avoid lots of errors that might occur due to conflicts and confusions regarding path symbols like: /, //, \. This problem occurs more often than you’d imagine and it can be very frustrating to troubleshoot. Just use r in front and path problems no more!
See demonstration below:
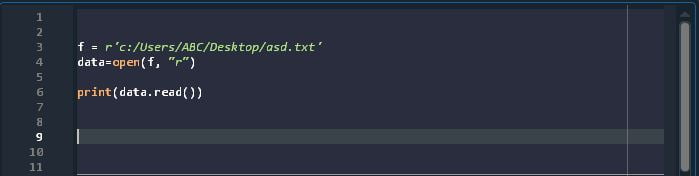
Also, in some cases you might need to use double back slashes instead of forward slashes. This is because different systems use different path structures. When working with a script that communicates with Windows OS for example, you’ll need to use “\\“
4) Big Number Readability: (Millions and Billions)
you can separate zeros with underscore (_)
This one is a potential favorite for teachers, scientists, finance quants, accountants, quantum physicists, actuaries, traders and all the rest of the big number people.
Underscore _ can be used to separate zeros in Python and this will make big numbers more readable while mathematically it won’t affect the syntax so you can still carry out arithmetic operations as normal:
This makes big numbers way more readable when needed.
print(1_000_000)
print(1_000_000 +1)
1000000
1000001
5) Let the code draw: (Python Turtle Drawing)
turtle
it’s worth mentioning in case you might have missed it. Turtle is a drawing library that works with Python codes. Creating patterns with turtle can be super intuitive in learning Python.
We have some extensive tutorials that are guaranteed to step up your Python skills especially regarding programming fundamentals. Here are a couple of articles to check:
The ultimate Python Turtle Tutorial for learning main Python concepts.
6) Chain Operators: (Larger Than and Smaller Than)
You can chain comparison operators in Python as following:
n = 10
result = 1 < n < 20
distance = 100
checker = 50 < distance < 250
print(checker)
True
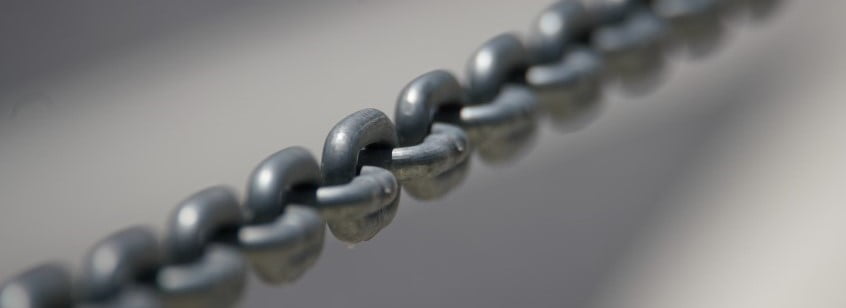
7) Pretty Print: (Pprint)
print function of Python has some pretty cool features already. But sometimes you might need something just a bit more powerful. pprint offers some structured printing opportunities when your data is more structured and nested (as it occurs with most web queries, web crawling, database results etc.)
Here is an example:
(data is taken from a geopy result as demonstrated in #74 in this article.)
data = {'place_id': 259174015, 'licence': 'Data © OpenStreetMap contributors, ODbL 1.0. https://osm.org/copyright',
'osm_type': 'way', 'osm_id': 12316939, 'boundingbox': ['42.983431438214', '42.983531438214', '-78.706880444495',
'-78.706780444495'], 'lat': '42.983481438214326', 'lon': '-78.70683044449504', 'display_name': '''230, Fifth Avenue,
Sheridan Meadows, Amherst Town, Erie County, New York, 14221, United States of America''',
'class': 'place', 'type': 'house', 'importance': 0.511}
pprint.pprint(data, indent=3)
{ 'boundingbox': [ '42.983431438214',
'42.983531438214',
'-78.706880444495',
'-78.706780444495'],
'class': 'place',
'display_name': '230, Fifth Avenue,\n'
' Sheridan Meadows, Amherst Town, Erie County, New '
'York, 14221, United States of America',
'importance': 0.511,
'lat': '42.983481438214326',
'licence': 'Data © OpenStreetMap contributors, ODbL 1.0. '
'https://osm.org/copyright',
'lon': '-78.70683044449504',
'osm_id': 12316939,
'osm_type': 'way',
'place_id': 259174015,
'type': 'house'}
8) Getting rid of unwanted characters: (left strip, right strip and just strip)
You can get rid of whitespaces or any specific character using strip methods in Python. You can use either plain strip for both sides, lstrip for the left side and rstrip for the right side only.
str="+++World Cup+++"
str.strip()
print(str)
World Cup
9) Merging strings: (join method)
You can use join method to combine strings. Very useful indeed.
lst="Nepal, Bhutan, Korea, Vietnam"
str=",".join(lst)
print(str)
Nepal, Bhutan, Korea, Vietnam
10) Versatile Arithmetic Operators: (Multiply Lists & Add Strings)
Everybody can do arithmetic with numbers but Python can do arithmetics with non-numbers too.
You can add strings and lists with arithmetic operator + in Python.
asd + asd
= asdasd
str1="hey"
str2=" there"
str3="\n"
print(str1+str2)
print(str3*5)
hey there
\n\n\n\n\n
11) Floor Division: (Keep the change)
When we speak of division we normally mean (/) float division operator, this will give a precise result in float format with decimals.
For a rounded integer result there is (//) floor division operator in Python. Floor division will only give integer results that are round numbers.
print(1000 // 400)
print(1000 / 400)
2
2.5
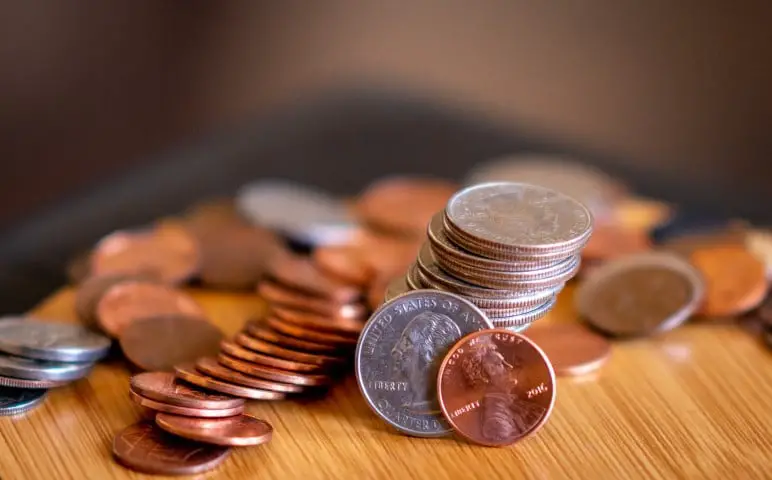
12) Negative Indexing: (Accessing from the end)
In Python you can use negative indexing. While positive index starts with 0, negative index starts with -1.
Here is a demo:
(last one is from index -1 to -4 with steps of -1), see below for more information.
name="Crystal"
print(name[0])
print(name[-1])
print(name[0:3])
print(name[-1:-4:-1])
C
l
Cry
lat
If you’re confused about this format or negative indexing as well as Python slice notation with negative steps here are 2 great resources to learn and practice.
Python Slice Notation Exercises
13) Break up strings: (Split Method)
Split function is a must know from the early on. Whether it’s data science or website development or database applications or just simple Python practicing, split function is one powerful function and it makes working with strings so much easier.
Its use is simple:
str = "Hello World"
a = str.split(" ")
print (a)
["Hello", "World"]
14) When the code is too fast: (Make Python Sleep)
Sometimes you want your code to execute slowly. You might want to demonstrate something or there might be steps that require little breaks. sleep method of time library is perfect for that.
Make secs any integer representing seconds:
import time
time.sleep(secs)
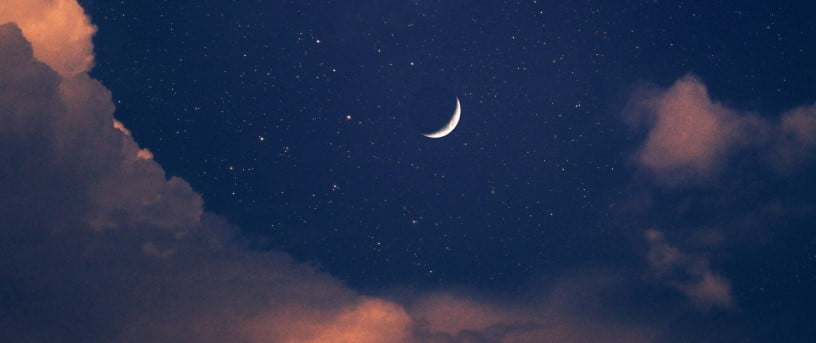
15) Reverse data: (Slice Notation)
8- Reversing through slicing
str="Californication"
a=str[::-1]
print(a)
noitacinrofilaC
16) Reverse data: (Reversed Function & Reverse Method)
Reversed function and reverse method can only be used to reverse objects in Python. But there is a major difference between the two:
- reversed function can reverse and iterable object and returns a reversed object as data type.
- reverse method can only be used with lists as its a list method only.
Check out 3 examples below:
1-
lst=["earth","fire","wind","water"]
lst.reverse()
print(lst)
['water', 'wind', 'fire', 'earth']
2-
lst=["earth","fire","wind","water"]
a=reversed(lst)
print(a)
print(list(a))
<reversed object at 0x000001C247364C88>
['water', 'wind', 'fire', 'earth']
3-
str="Californication"
a=reversed(str)
print(("".join(a)))
noitacinrofilaC
17) Multi Assignments: (One-Liner)
This one is self explanatory but still good to be aware of:
a = [1000, 2000, 3000]
x, y, z = a
print(z)
3000
18) Code Sections: (Spyder)
6- #%%
Creating Code Sections in your IDE (Spyder)
Are you coding in Spyder? Use this command to create subsections in your code and you can run each section with Ctrl+Enter.
This trick almost converts your Spyder IDE to a Notebook, very cool trick for practicing, experimenting and learning or teaching purposes.
If you’d like to install Spyder a free open source Python IDE that we recommend, it comes with Anaconda click here to see how to install Anaconda.
See demonstration below:
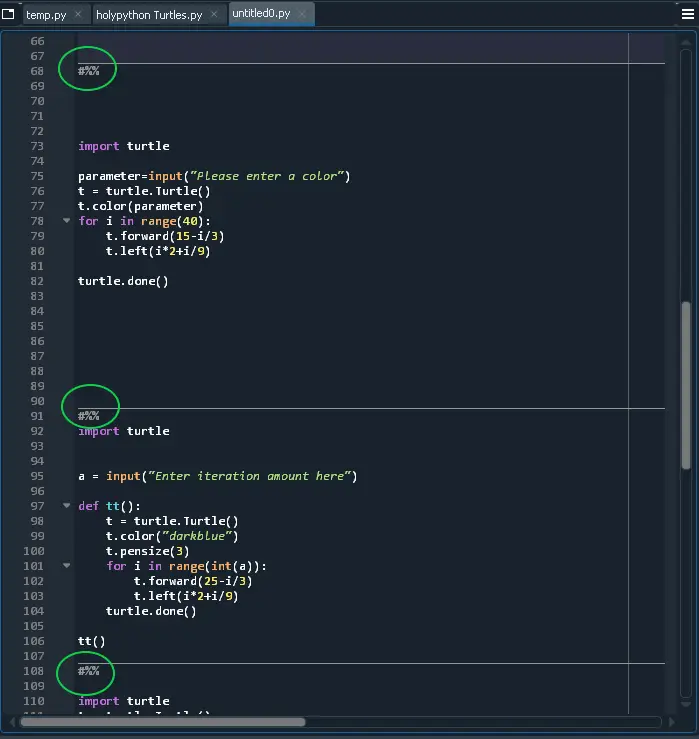
19) Remove Duplicates: (set function)
Most straightforward approach to removing duplicates in a list is probably using the set() function.
Set in Python is a datatype that’s sort of between lists and dictionaries.
It takes unique values only, it’s mutable and like dictionaries it’s unordered. Here is an example:
lst=[1,2,2,2,2,3,4,4,5,6,7]
a=set(lst)
print(a)
{1, 2, 3, 4, 5, 6, 7}
The new object will have a “set” type.
print(type(a))
<class 'set'>
If you want to overcome the type conversion and keep your data as a list just add a list function as below and it will stay as a list after set function removes all the duplicates:
lst=[1,2,2,2,2,3,4,4,5,6,7]
a=list(set(lst))
print(a)
print(type(a))
{1,2,3,4,5,6,7} <class 'list'>
20) Python Working Directory: (os.getcwd())
2- This one is so helpful when needed. Sometimes you may need to identify or double check where your Python is working (directory wise)
get working directory with getcwd() method of os library:
import os
dirpath = os.getcwd()
'C:\\Users\ABC>
This information can be important sometimes when you’re coding and dealing with data and/or files etc.
For instance: you can use only file names to work with files in your working directory instead of their full file path names.
20) Python Working Directory: (os.chdir())
So what if you’re not happy with the current working directory? Don’t worry, you can use the same Python library named os to change the working directory.
change working directory with chdir() method of os library:
ps(don’t forget to use r letter in front of your path to avoid path notion conflicts.)
import os
os.chdir(r'c:/Users/ABC/Desktop')
print(os.getcwd())
'C:\\Users\ABC\Desktop>'
22) Printing libraries: (To get their directiories)
73- print a library
Have you ever tried printing a library name directly in Python? What will happen is, it will tell you the location where library file is located. Pretty cool and such a Python feature.
import pandas
print(pandas)
<module 'pandas' from 'C:\\Anaconda3\\lib\\site-packages\\pandas\\__init__.py'>
23) Membership Operators: (in & not in)
Makes it very convenient to find out if a subgroup exists in a data object
example with in
lst = "10, 50, 100, 500, 1000, 5000"
for i in lst:
if i == str(0):
print(i, end="|")
0|0|0|0|0|0|0|0|0|0|0|0|
example with not in
lst = list(range(100))
if 500 not in lst:
print("Value is out of range")
Value is out of range
24) Advanced Print: (sep parameter)
Do you want to print multiple values with a user-defined separater? You can use print’s sep parameter for that.
str1="bluemoon"
str2="geemayl.com"
print(str1, str2,sep="@")
bluemoon@geemayl.com
25) Advanced Print: (end parameter)
Python’s print function has a \n new line character at the end by default.
But, sometimes, you just don’t want it to enter a new line after print or better yet you might want to add a different character to the end of each print function.
end= parameter is perfect for that. Here is an example:
print("one" , end ="," )
print("two", end =",")
print("three")
one, two, three
26) Triple quotes: (Multiline Strings)
”’some text here”’
Triple quotes are a solution to a common complication when working with strings.
Python will let you create multi-line strings as long as you use triple quotation marks, it works with both single quotes and double quotes as long as you use 3 of them.
Here is a simple example:
a = """Here is a nice quote from George Orwell's 1984:
Under the spreading chestnut tree I sold you and you sold me:
There lie they, and here lie we Under the spreading chestnut
tree."""
print(a[-15:-1])
chestnut
tree
27) List Comprehension: ()
List comprehension is a very cool way to build lists in 1 line.
You can include conditional statements, lambda, functions, loops all in 1 line of list comprehension code. No wonder why it’s praised so much.
Try these list comprehension exercises and you’ll see how useful and addictive they can be.
28) Dict Comprehension: ()
Dict comprehension is lesser known than list comprehension but that doesn’t mean it’s less impressive. You can build dictionaries from almost any structure and manipulate different combinations of dictionary keys and values with this Python structure.
Here are some dict comprehension exercises as well in case you’d like to practice them.
29) Partial assignments: (List Unpacking)
Do you want to assign one or more elements of a list specifically and assign all the remains to something else? Easy with Python.
Check out this syntax that makes use of * unpacking notation in Python:
lst = [10, 20, 30, 40]
a, *b = lst
print(a)
print(b)
10
[20,30,40]
Here is another example, basically variable with the star (*) gets assigned to the leftovers.
lst = ["yellow", "gray", "blue", "pink", "brown"]
a, *b, c = lst
print(a)
print(b)
print(c)
"yellow"
["gray", "blue", "pink"]
"brown"
30) Ordered Dictionaries: (from collections)
Python’s default dictionary data structure doesn’t have any index order. You can think of key-value pairs as mixed items in a bag. This makes dictionaries very efficient to work with. However, sometimes you just need your dictionary to be ordered.
No worries, Python’s collections library has a module named OrderedDict that does just that.
import collections
d = collections.OrderedDict()
d[1] = 100
d[2] = 200
print(d)
OrderedDict([(1, 100), (2, 200)])
31) Permutations: (from itertools)
Whether it’s betting with your friends, calculating a sophisticated mathematical equation, reading Adam Fawer’s Improbable or evaluating your chances before a Vegas trip; you never know when you might need a couple of permutation calculations.
Thanks to Python you don’t have to go through the tedious arithmetics of permutations and you can get it done with a simple piece of code.
Check out this making use of permutations method from itertools library:
First print shows to total amount of different probabilities, second print demonstrates all the possible events.
import itertools
lst = [1,2,3,4,5]
print(len(list((itertools.permutations(lst)))))
for i in itertools.permutations(lst):
print (i, end="\n")
120
(1, 2, 3, 4, 5)
(1, 2, 3, 5, 4)
(1, 2, 4, 3, 5)
(1, 2, 4, 5, 3)
(1, 2, 5, 3, 4)
(1, 2, 5, 4, 3)
(1, 3, 2, 4, 5)
(1, 3, 2, 5, 4)
(1, 3, 4, 2, 5)
(1, 3, 4, 5, 2)
(1, 3, 5, 2, 4)
(1, 3, 5, 4, 2)
(1, 4, 2, 3, 5)
(1, 4, 2, 5, 3)
(1, 4, 3, 2, 5)
(1, 4, 3, 5, 2)
(1, 4, 5, 2, 3)
(1, 4, 5, 3, 2)
(1, 5, 2, 3, 4)
(1, 5, 2, 4, 3)
(1, 5, 3, 2, 4)
(1, 5, 3, 4, 2)
(1, 5, 4, 2, 3)
(1, 5, 4, 3, 2)
(2, 1, 3, 4, 5)
(2, 1, 3, 5, 4)
(2, 1, 4, 3, 5)
(2, 1, 4, 5, 3)
(2, 1, 5, 3, 4)
(2, 1, 5, 4, 3)
(2, 3, 1, 4, 5)
(2, 3, 1, 5, 4)
(2, 3, 4, 1, 5)
(2, 3, 4, 5, 1)
(2, 3, 5, 1, 4)
(2, 3, 5, 4, 1)
(2, 4, 1, 3, 5)
(2, 4, 1, 5, 3)
(2, 4, 3, 1, 5)
(2, 4, 3, 5, 1)
(2, 4, 5, 1, 3)
(2, 4, 5, 3, 1)
(2, 5, 1, 3, 4)
(2, 5, 1, 4, 3)
(2, 5, 3, 1, 4)
(2, 5, 3, 4, 1)
(2, 5, 4, 1, 3)
(2, 5, 4, 3, 1)
(3, 1, 2, 4, 5)
(3, 1, 2, 5, 4)
(3, 1, 4, 2, 5)
(3, 1, 4, 5, 2)
(3, 1, 5, 2, 4)
(3, 1, 5, 4, 2)
(3, 2, 1, 4, 5)
(3, 2, 1, 5, 4)
(3, 2, 4, 1, 5)
(3, 2, 4, 5, 1)
(3, 2, 5, 1, 4)
(3, 2, 5, 4, 1)
(3, 4, 1, 2, 5)
(3, 4, 1, 5, 2)
(3, 4, 2, 1, 5)
(3, 4, 2, 5, 1)
(3, 4, 5, 1, 2)
(3, 4, 5, 2, 1)
(3, 5, 1, 2, 4)
(3, 5, 1, 4, 2)
(3, 5, 2, 1, 4)
(3, 5, 2, 4, 1)
(3, 5, 4, 1, 2)
(3, 5, 4, 2, 1)
(4, 1, 2, 3, 5)
(4, 1, 2, 5, 3)
(4, 1, 3, 2, 5)
(4, 1, 3, 5, 2)
(4, 1, 5, 2, 3)
(4, 1, 5, 3, 2)
(4, 2, 1, 3, 5)
(4, 2, 1, 5, 3)
(4, 2, 3, 1, 5)
(4, 2, 3, 5, 1)
(4, 2, 5, 1, 3)
(4, 2, 5, 3, 1)
(4, 3, 1, 2, 5)
(4, 3, 1, 5, 2)
(4, 3, 2, 1, 5)
(4, 3, 2, 5, 1)
(4, 3, 5, 1, 2)
(4, 3, 5, 2, 1)
(4, 5, 1, 2, 3)
(4, 5, 1, 3, 2)
(4, 5, 2, 1, 3)
(4, 5, 2, 3, 1)
(4, 5, 3, 1, 2)
(4, 5, 3, 2, 1)
(5, 1, 2, 3, 4)
(5, 1, 2, 4, 3)
(5, 1, 3, 2, 4)
(5, 1, 3, 4, 2)
(5, 1, 4, 2, 3)
(5, 1, 4, 3, 2)
(5, 2, 1, 3, 4)
(5, 2, 1, 4, 3)
(5, 2, 3, 1, 4)
(5, 2, 3, 4, 1)
(5, 2, 4, 1, 3)
(5, 2, 4, 3, 1)
(5, 3, 1, 2, 4)
(5, 3, 1, 4, 2)
(5, 3, 2, 1, 4)
(5, 3, 2, 4, 1)
(5, 3, 4, 1, 2)
(5, 3, 4, 2, 1)
(5, 4, 1, 2, 3)
(5, 4, 1, 3, 2)
(5, 4, 2, 1, 3)
(5, 4, 2, 3, 1)
(5, 4, 3, 1, 2)
(5, 4, 3, 2, 1)
Here is a little twist if you’d like to see all the different numbers that are possible to generate with those 5 integers:
import itertools
lst = [1,2,3,4,5]
print(len(list((itertools.permutations(lst)))))
for i in itertools.permutations(lst):
print (''.join(str(j) for j in i), end="|")
12345|12354|12435|12453|12534|12543|13245|13254|13425|13452|13524|13542|14235|14253|14325|14352|14523|14532|15234|15243|15324|15342|15423|15432|21345|21354|21435|21453|21534|21543|23145|23154|23415|23451|23514|23541|24135|24153|24315|24351|24513|24531|25134|25143|25314|25341|25413|25431|31245|31254|31425|31452|31524|31542|32145|32154|32415|32451|32514|32541|34125|34152|34215|34251|34512|34521|35124|35142|35214|35241|35412|35421|41235|41253|41325|41352|41523|41532|42135|42153|42315|42351|42513|42531|43125|43152|43215|43251|43512|43521|45123|45132|45213|45231|45312|45321|51234|51243|51324|51342|51423|51432|52134|52143|52314|52341|52413|52431|53124|53142|53214|53241|53412|53421|54123|54132|54213|54231|54312|54321|
You can see all the other cool modules from itertools library on this Official Python page of itertools.
32) Factorial: (user function)
How about factors of a number? This can easily be achieved by using the % modulus operator of Python.
In a range from 1 up to the number we’re searching factors for, if any number can divide the main number without any remainders, that means its a factor of our number. Here is the idea in code:
n = 159
for i in range(1, n + 1):
if f % i == 0:
print(i)
1
3
53
159
33) clear console: (Spyder)
This one is for Spyder users but you can imagine there is a command to clear all IDE consoles.
Simply type clear on the console and your console will be cleared.
Note: type clear in the console (where you get outputs, or values get printed) instead of where you code (the editor).
34) N largest & N smallest: (Standard heapq library)
Sometimes max and min functions might not cut it for you when you need multiple max or multiple min values from a set of values.
Check out heapq library that makes getting n largest or n smallest values much easier than usual techniques:
It works with the floats too
import heapq
grades = [5, 78, 6, 30, 91, 1005.2, 741, 1.9, 112, 809.5]
print(heapq.nlargest(2, grades))
print(heapq.nsmallest(5, grades))
[1005.2, 809.5]
[1.9, 5, 6, 30, 78]
35) Make it immutable: (frozenset)
frozenset() function allows lists to be immutable.
On some occasions you might have started your program with a list instead of tuples because of the conclusion that mutable data structure is more suitable for the project. But things change, projects evolve and ideas are known to change route. Now you decide that you needed immutable structure but it seems to late to undertake the tedious work of converting your lists? No worries. frozenset() will make it a breeze.
lst = ["June", "July", "August"]
lst[0]="May"
print(lst)
['May', 'July', 'August']
i_lst=frozenset(lst)
print(i_lst)
i_lst[0] = "June"
TypeError: 'frozenset' object does not support item assignment
Bonus) Short library names: (import as)
Libraries are Python’s strong suit. Whether it’s external libraries or default libraries there is so much to discover and make use of.
That also means they come with all sorts of names. When you’re using a library frequently in a code it can get tedious to type the full name while using library’s methods.
To help with that you can import libraries as with a more convenient name. Here are some examples:
import matplotlib as mpl
import pandas as pd
import numpy as np
import statsmodels as sm
import tensorflow as tf
import seaborn as sb
This is the end of our Beginner Python Tips.
You can access the continuation of 100 Python Tips through the links below: