- Logistic Regression History
- 29 July 2020
- by HolyPython
Theoretical Foundations
Genius of a Belgian Activist Mathematician
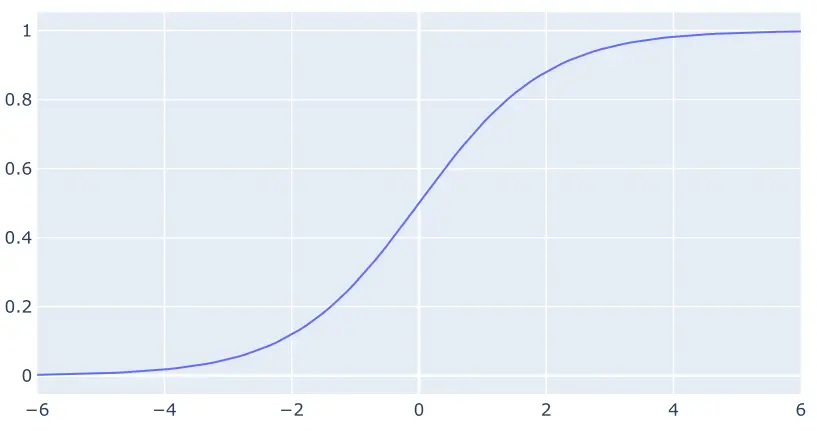
Ancient Times
First appearance of Logistic Function is seen in early 19th century.
Pierre François Verhulst published logistic function in 1838 under the name: Correspondance mathématique et physique.
Verhulst was a Belgian mathematician and lived a short life between 1804 and 1849 in Brussels.
His book was published by the support and supervision of Belgian mathematician and polymath Adolphe Quetelet who was also his mathematics teacher.
Verhulst had wealthy parents and he had an interesting life although unfortunately he wasn’t blessed with good health. The real reason for his health complications is unknown due to primitiveness of medicine technology in his days but the culprit for his bad health is suspected to be tuberculosis.
I think it is also fair to say that Verhulst was sort of an activist. When he went to Rome to profit from the sunny weather and hopefully improve his health, he has made a new constitution draft and discussed it with cardinals and exiled Queen of Holland, Hortense de Beauharnais and convinced her to submit it to the Pope. His criticism wasn’t taken lightly back then and he was eventually deported back to Belgium only to encounter Belgian revolution this time.
In 1845 he published a more detailed version of logistic function: “Recherches mathématiques sur la loi d’accroissement de la population”
Verhulst developed logistic function to model population growth initially but it has been a major model since then and is used in every field of science and technology today.
J.S. Cramer has a great paper dedicated to the history of logistic regression which can be accessed here: “The Origins of Logistic Regression (2002)”
After Verhulst’s initial discovery of logistic function, the most notable discoveries were the probit model, developed by Chester Ittner Bliss in 1934 and maximum likelihood estimation by Ronald Fisher in 1935.
Throughout the late 19th century and early 20th century logistic function has been rediscovered a few more times.
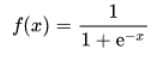
Probit vs Logit
In 1943 Wilson and Worcester used logistic model in bioassay which was the first known application of its kind.
Joseph Berkson, though, really took logistic model by the heart and named it logit following the probit terminology for many following years. Although logit wasn’t going to be accepted initially, deemed as inferior to the probit model, future was going to unfold more positively for logit.
After numerous contributions in 1940s and 1950s Cox was going to come up with multinomial logit model.
In 1966 Cox introduced multinomial logit model which became a step up for the logistic regression applications with the logit model.
In 1973 McFadden made a connection between multinomial logit model and discrete choice theory and the rest is history. He was working as a consultant in California during his discovery and 27 year later he was awarded Nobel prize for his contribution in 2000.
Today Logistic Regression is regarded as one of the most common and useful machine learning algorithm.
Probit and logit are models used in regression. While logit (also aka log-odds) is inverse of the logistic function, probit is a model that uses cumulative distribution function.
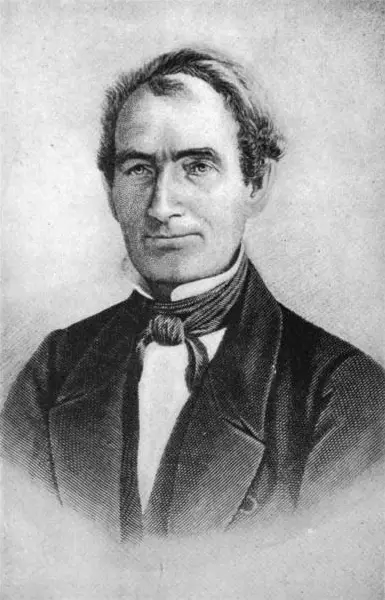
Finishing Thoughts
Logistic Regression is a really sophisticated machine learning model with theoretical foundation and mathematical roots. Its roots definitely go back to Verhulst’s logistic function.
In that sense it’s easier to track the beginnings of Logistic Regression compared to something like “Decision Trees”.
I’ve really enjoyed the most about Belgian Statistician Verhulst’s short and colorful life. Considering how many times logistic function was independently rediscovered in the following years/decades, I think it’s safe to assume that sooner or later, logistic was bound to happen in mathematics literature.
Another interesting highlight of Logistic Regression history is how logit is initially dismissed for years and then becomes huge, eventually winning McFadden the Nobel Prize.
If you liked this history account, check out our tutorials about other Machine Learning Tutorials or feel free to dig deeper in Logistic Regression Model with Algorithm examples, step-by-step explanation and Visualization.